A model is a common language
Every tool used in IT operations speaks its own language. The collected data, the storage format and the API with which it is made available all differ from tool to tool. Even if the data describes the same IT system or the same event happening in the world, it will still be different. This presents AIOps products with a challenge. If your goal is to create a complete picture of an IT landscape, how do you do that if the data comes from different sources?
The answer is that you define a common language across those tools that allows you to know when they describe the same real world concept or event. This common language is called a model. It defines what types of "things" you can describe in the model, how each "thing" is identified and how the things relate to each other.
Once the information from each source is translated into this common language, you can suddenly combine, relate and compare information across all tools. It's like having a universal translator.
A model is great input for machine learning
Applying a model has additional benefits in the context of AIOps. When you've collected all the relevant information, the next step is to feed this into machine learning algorithms that will scour the data for hidden connections and correlations.
Supplying machine learning algorithms with structured data defined by a model works like jet fuel. Clearly defined data, aggregated, de-duplicated, well-structured and accessible in a machine-readable format allows machine learning to focus on the most critical activity: discovering valuable insights that help your business.
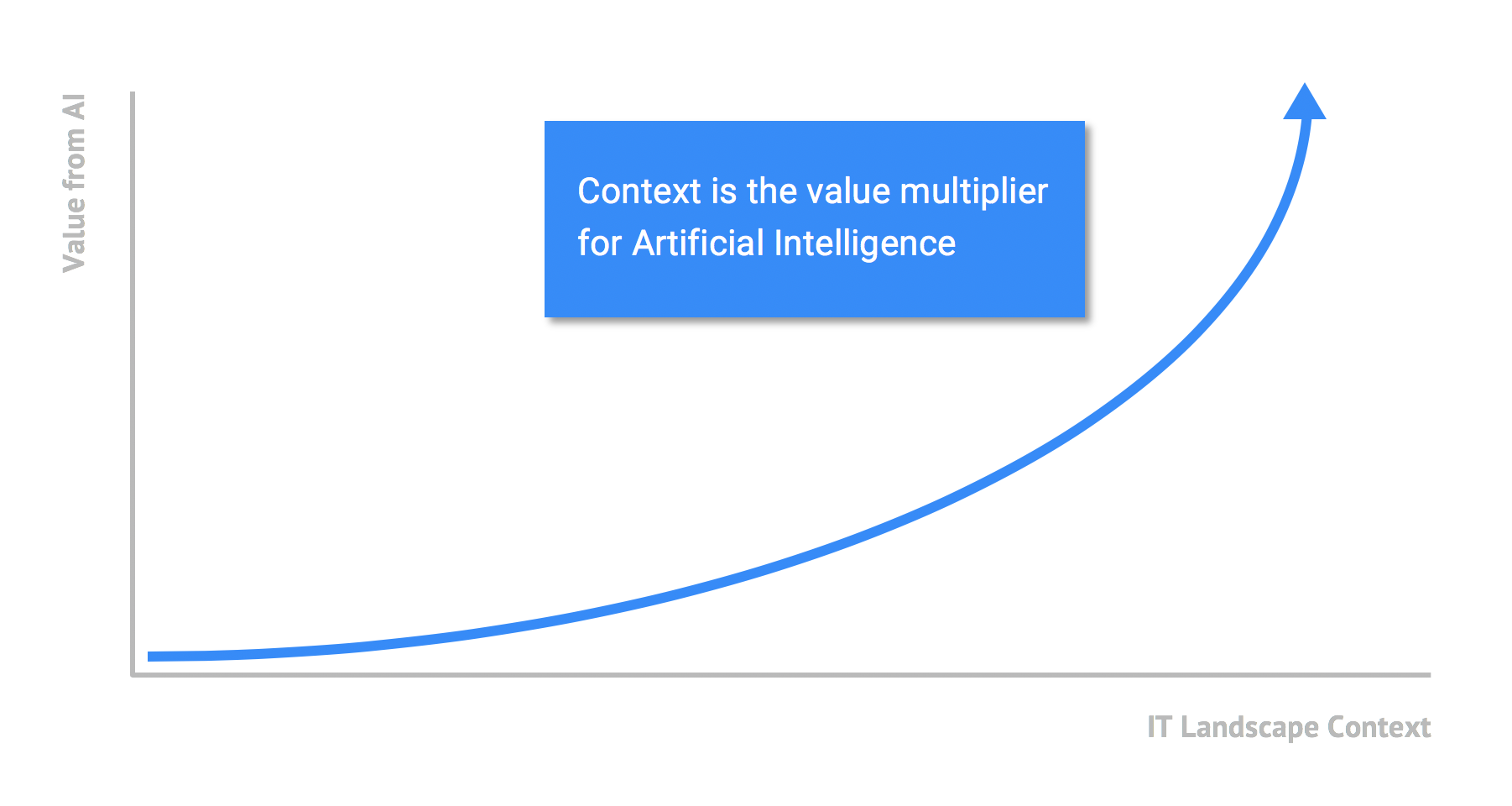
Many AIOps solutions use machine learning on raw, unstructured data, such as incoming events. These products count on the power of AI to discover structure in the data first, then attempt to find smart insights on top of that. The structure they attempt to find is what components exist in the landscape, how they relate to each other and what metrics exist that say something about their performance, exactly the information you can get from a model.
Using AI to discover structure is highly dependent on the data the algorithm receives. If certain parts of the landscape don't generate events, the AI won't know about them. And what if a discovered component stops generating events? Is it simply inactive or completely gone? Additionally, running machine learning algorithms on lots of data can be a time-intensive process. If all incoming data goes through an AI pipeline first, this could mean processing is delayed, resulting in failure alerts being delivered late.
A model frees you to ask your own questions
Finally, using data structured in a model gives you a unique opportunity: the ability to access the model programmatically and ask it your own questions, effectively giving you the ability to draw your own conclusions from the data. This frees users from only having access to the functionality the application exposes and allows them to think out of the box.
For example, suppose you want to know which applications in your landscape have suffered a performance degradation recently. Rather than navigate a complicated GUI, or correlating information from different tools, a model would allow you to ask a question like:
Which applications experienced a more than 10% drop in response time over the last 24 hours?
For each of the applications, give me a list of the changes that happened to surrounding application landscape last week?
A model exposed via a query language makes it possible to do this and any other query you can think of. The possibilities are literally endless.
Want to learn more?
Our own model is called the 4T Data Model. It ingests data from any source, then correlates, unifies and consolidates telemetry and trace data to create one visual topology of your entire IT environment - and tracks it all over time. The 4T Data Model, coupled with our AIOps capabilities give you deep insights into the health of your IT environment and also help you proactively prevent problems.
Based on research and conversations with enterprises from various industries we created the Observability Maturity Model. This model reflects the four stages of observability maturity. It will help you to understand your current observability maturity level and also inform you as to what steps you need to take to improve it.