In this blog, we will give a high-level explanation of these two dimensions and why they are so important for a future proof AIOps solution. Let’s start this blog with describing the problem AIOps platforms aim to solve.
No control over chaos
Organizations have started to introduce new principles like DevOps and SRE to deliver better and more reliable software faster. This accelerated innovation in hybrid and multi-cloud environments and the dynamics around the changes in infrastructure and networks create the urgent need to stay in control more than ever. As a result, IT operations have been adopting many different tools to monitor and manage every aspect of their complex IT landscape. This complexity can be defined across three dimensions:
Volume: Exploding growth in data volumes generated by the IT infrastructure and applications;
Variety: The increasing variety of data types generated by machines and humans (e.g. metrics, logs, events, traces, documents);
Velocity: The increasing velocity at which data is generated as well as the increasing rate of change within IT architectures.
Lack of insight despite all tools implemented
Although many efforts taken, current monitoring tools in the market do not cut across the multiple data types required for extracting holistic insights. Current monitoring systems are not equipped to handle today’s complex, scalable and dynamic IT landscapes. Too much work is left to be done manually, and the tools used by many IT organizations only address specific aspects of certain domains, such as applications, infrastructure or networks. These ‘domain-centric’ monitoring tools limit the IT operation’s ability to detect, diagnose and address performance issues and to predict potential impact on other critical parts inside the organization. Organizations should look at the entire system, rather than specific technologies and infrastructure layers.
AIOps platform – beyond human capabilities
To manage this increased complexity a data platform is needed that can handle the volume, variety and velocity of historical and streaming data. The solution lies within an AIOps platform. AIOps platforms enhance IT operations through greater insights by combining big data, machine learning and visualization:
Big data: the AIOps platform should be able to ingest a wide range of historical and streaming data types, including data beyond typical monitoring data like social media, or other business metrics, CMDBs, CI/CD tools, service registries, automation and incident management tools. This can also be described as the ‘openness’ of the data platform;
AI & Machine Learning: Based on the historical and streaming data ingested, the AIOps platform should be capable to automate human tasks by use of Artificial Intelligence and Machine Learning;
Visualization: the AIOps platform should be able to deliver actionable insights through platform visualizations, e.g. contextualization with topology, problem clustering and automated root cause analysis.
The importance of an open AIOps data platform
It’s all about building context. Context is the power IT organization have to relate data (e.g. events, metrics and logs) to one another, to accurately pinpoint problems, predict future incidents and understand which changes will impact business critical processes. The more and varying data is ingested into an AIOps platform, the more value the algorithms can deliver in terms of useful insights. Moreover, analyzing changes in your entire IT landscape from different perspectives will result in more accurate outcomes. Therefore, these outcomes have more value to the user.
Domain-centric vs. Data-agnostic vs. Value
As described, the openness of an AIOps platform plays a crucial role in the potential value the current AI capabilities deliver to the user. Let’s focus on these two dimensions and their outcome:
The openness of the data platform: this is the level in which the platform is capable of delivering a broad set of use cases towards the future. Within this dimension, we see two types of vendors. Data-agnostic vendors are very open and can ingest a variety of data sources and data types. Domain centric vendors have a more closed character and focus on a limited set of data sources and data types.
AI & machine learning capabilities:
the degree in which current AIOps vendors apply AI and Machine learning to deliver actionable insights to help organizations boost team productivity in complex IT landscapes.
Outcome: Value from AI and machine learning for the user.
How the two dimensions relate to the potential value from AI capabilities can easily be visualized with the quadrant below. It’s not “Magic” like the to come AIOps Quadrant from Gartner, but it does deliver high level insight.
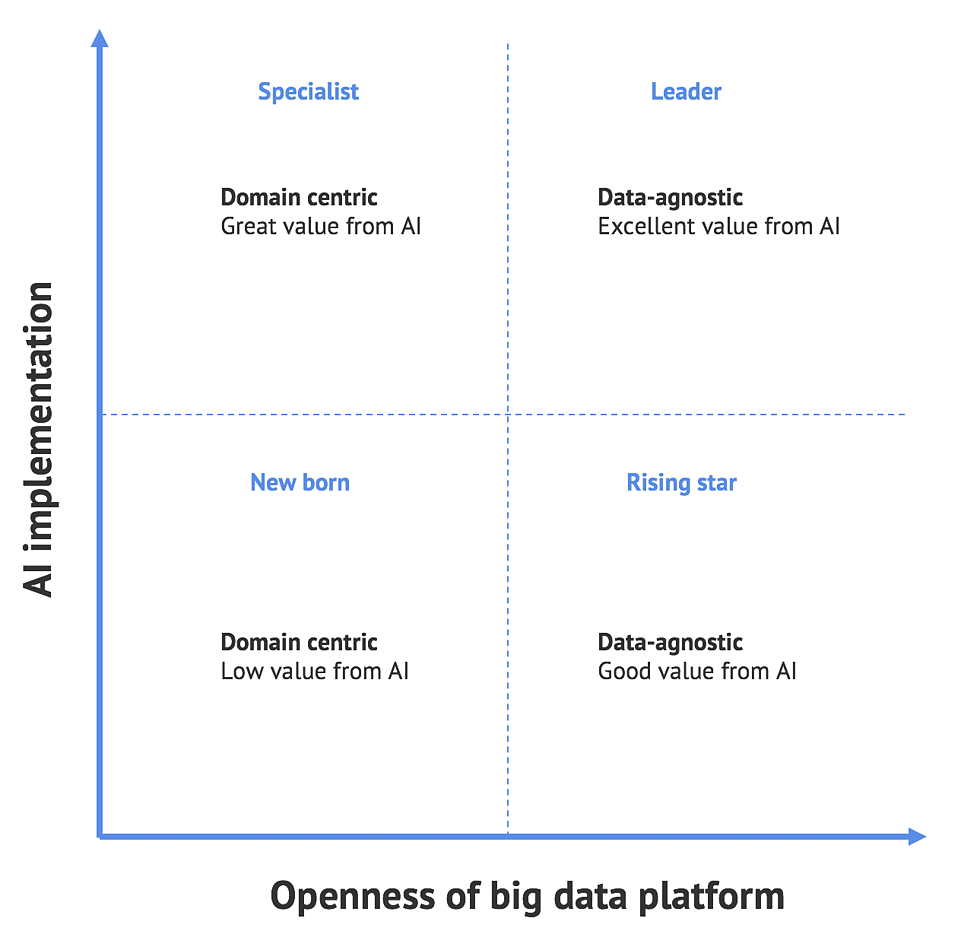
The four quadrants:
Leader: leaders offer a data-agnostic data platform, capable of ingesting many different data sources and data types and have great AI and machine learning capabilities in place to drive service delivery outcomes. As the algorithms are fueled by a very broad range of data types and data sources, the potential value from the AI is excellent. Currently, there are no vendors yet in this space.
Specialist: specialists work with specific data sources and data types. These are vendors that have great AI in place, but the AI capabilities are fueled by limited data sources and focus on specific data types. Vendors in this quadrant are e.g. Moogsoft (event focus), Splunk (logs focus) and Dynatrace (traces focus).
Rising star: rising stars have invested greatly in the openness of their data platform and can ingest almost any type of data. However, these vendors have had less focus on implementing AI & and Machine learning. Therefore, the current value is lower than that from the specialists. However, as the platform is engineered as highly data-agnostic, the potential of the AI capabilities and the value it will deliver is enormous. Vendors like StackState are positioned in this quadrant.
New born: new borns are obviously new in the AIOps market. Most of the time, they start working up towards the Specialist position and then work towards the Leader position.
Two trends in the current AIOps market
As the future of IT Operations lies within data-agnostic AIOps platforms, we see two trends:
Vendors in the specialist quadrant are slowly moving to the right. This means these vendors are re-engineering the core of their data platform to be able to ingest a wider range of data types and data sources. E.g. vendors that have been historically focused on collecting and analyzing events, are developing new capabilities around collecting and analyzing metrics, and are also developing techniques for better understanding customer topologies.
On the other hand, we also see that vendors in the rising star quadrant are moving up fast. This means these vendors already have their data-agnostic data platform in place and are now setting up specialized AI and Machine learning teams to deliver excellent AI value out of all historical and streaming data collected.
Which starting position is better? Future will tell. However, re-engineering the core of a complex product is a difficult challenge, which requires long and determined efforts. It is probably way more difficult than engineering new AI and Machine learning capabilities on top of an already solid data-agnostic AIOps platform.
About StackState’s AIOps platform
StackState analyzes metrics, logs, events and other data, including data beyond typical monitoring data, like business metrics from Google Analytics, CMDBs, CI/CD tools, service registries, automation and incident management tools. StackState uses the data it collects to learn about dependencies, allowing it to build a topology of a user's IT environment in real time. Customers can use the StackState agent to collect metrics, or they can rely on data collected by third-party monitoring and logging tools that they already have in use. This data is also used as fuel for StackState’s AI and machines learning capabilities, to instantly assist teams in discovering the root cause when incidents strike. Learn more here.